- HOME
- More
- Technology
- How to train your AI
How to train your AI
- Last Updated : March 12, 2024
- 100 Views
- 3 Min Read
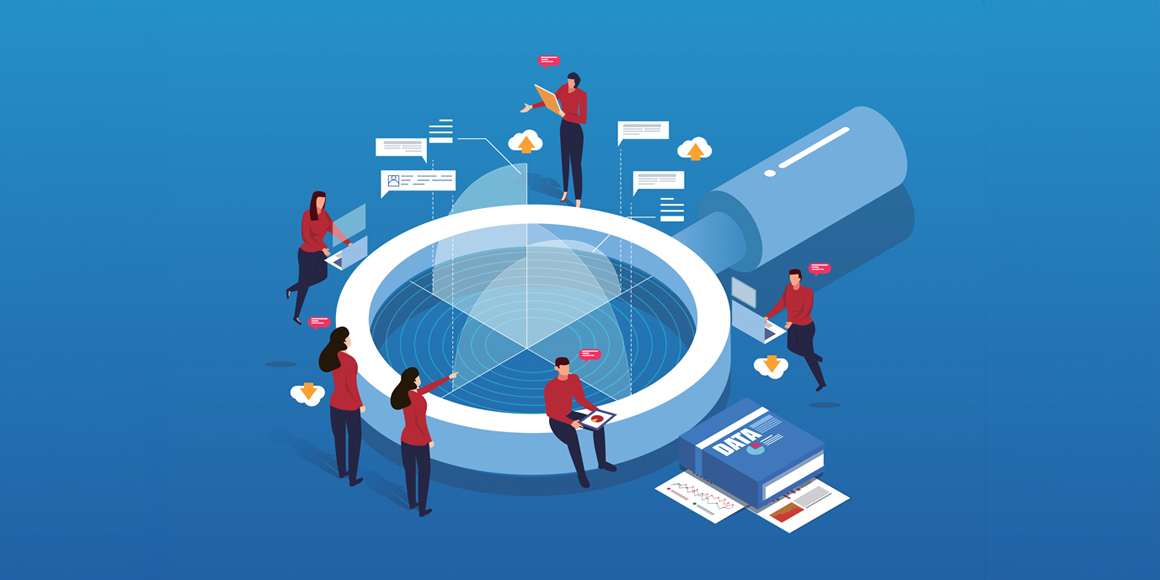
Though AI and related technologies have been around for a long time, the advent of accessible and easy-to-use applications, such as GenAI and LLMs, have created a new wave of interest. Today, technology vendors are including both traditional and new-gen AI capabilities wherever possible, and organizations are using them for whichever business functions they're applicable.
If the first pillar of AI tools is the underlying technology itself, the second pillar is the data used to train them. Therefore, in order to enable these tools to deliver the best possible insights for your organization, it's essential that you provide them with data that possesses certain qualities. In this article, we'll look at what these qualities are.
Accuracy
This is the first and most important quality that the data must possess, because you're ultimately going to use AI tools to make decisions. If the tool is fed with inaccurate or outdated data, you stand the risk of making incorrect decisions—so make sure to follow best practices, such as data validation and cleansing, so that the tool uses only accurate and clean data.
Completeness
This is the next important quality, because massive chunks of data usually contain variables and patterns that can evade the human eye. The more comprehensive and detailed the data is, therefore, the more correlations and insights your AI tool can deliver.
Since it's also likely that you'll be using the tool to aid as many different business functions as possible, that's all the more reason to ensure the data contains varied data points. For instance, if you're adding transactional data, it'd be prudent to include all details, such as date, time, location, browser (for online transactions), payment mode, referral (if any), purchase value, date of previous purchase, and so on.
Consistency
The next quality that the data must possess is consistency, both in terms of structure and update frequency. Before feeding the data to the tool, make sure that all items possess entries for all data points. Handling this data becomes a lot more challenging if you're a large enterprise that uses different software for different purposes. To take a simple example, one tool might be recording time on a 12-hour clock, whereas another might be recording on a 24-hour clock. Inconsistencies such as these might confuse the AI tool and lead to confusing or unactionable insights.
Therefore, it's important to spend plenty of time reviewing all the data points and standardizing them. Also, make sure that you keep feeding data to the tool at regular intervals. This way, the tool keeps receiving updated data and learns more about your organization and your customers.
Objectivity
One of the biggest criticisms that GenAI tools like ChatGPT and DALL.E have faced is the bias evident in their outputs. For instance, OpenAI has written about how they identified that the tool might be generating biased content and what steps they took to mitigate it. More recently, at the end of an experiment, ChatGPT itself admitted that it could potentially be creating content with racial bias.
This points to the need to ensure that AI tools receive objective data that's free from biases. Therefore, keep in place checks and measures that regulate what kind of data you feed to the AI tools you use. Make sure to include varied representations and diversity as parameters while validating your data.
A word of caution
As you incorporate the aforementioned qualities into your data, make sure that you adhere to privacy and security best practices at all times. Be wary of the data sources you use to train your AI models and make sure that critical data from your organization doesn't get out.
Back in the early 2010s, Target made a serious privacy faux pas when using data for predictive analytics. Over a decade has passed since then, and organizations know better now. Still, this incident can serve as a reminder to take privacy seriously. Moreover, the cost of security and privacy lapses today can be debilitating to organizations thanks to stringent privacy and data protection laws across the globe. The promise and potential of AI can be exciting, but it's essential that you remain cautious and pragmatic with how you use it.