AI bias: Challenges, causes, and how to overcome them
- Last Updated : February 5, 2025
- 144 Views
- 5 Min Read
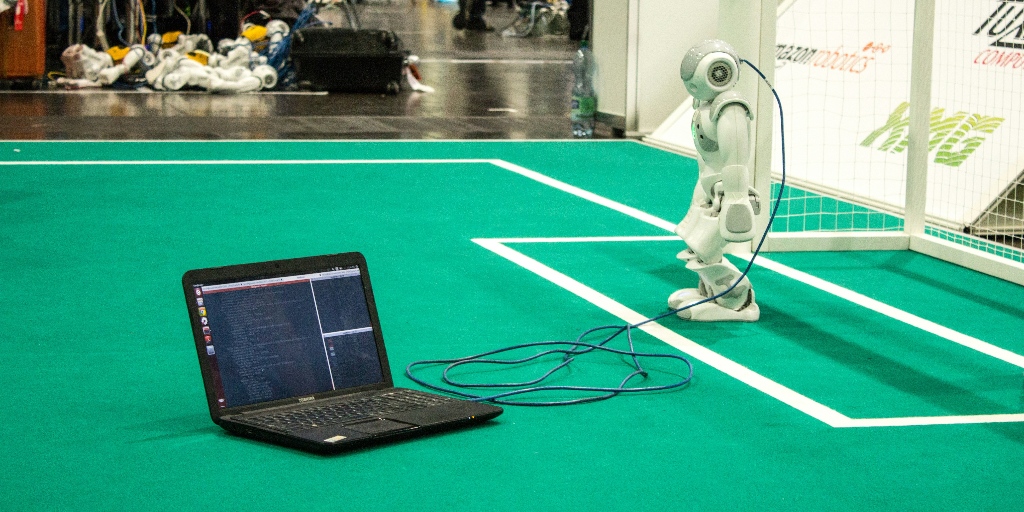
AI is changing the way we work and live, from improving healthcare and finance to reshaping entertainment and transportation. However, as AI systems become more integrated into our daily lives, there’s an increasing concern about one critical issue: AI bias.
AI bias refers to systematic errors in an AI model’s predictions or behaviour. These errors occur due to biased data or flawed algorithms. These biases can lead to unfair, inaccurate, or harmful outcomes, particularly when it comes to marginalised groups or sensitive areas like hiring, criminal justice, or lending.
In this blog, we’ll explore what AI bias is, its causes, its potential consequences, and most importantly, how to mitigate it.
What is AI bias?
AI bias occurs when an AI system makes decisions that are systematically prejudiced due to various factors, such as:
- Data bias: AI models are only as good as the data they’re trained on. If the data contains biased patterns—whether due to historical inequality, societal stereotypes, or incomplete representation—the AI model will learn these biases and carry them forward in its predictions.
- Algorithmic bias: Sometimes, the algorithms themselves are designed in a way that inadvertently favours certain groups or outcomes. Even well-intentioned algorithms can create biased results if they are not carefully tested and reviewed.
- Human bias: The biases of the people who design, train, and implement AI systems can also influence how these systems function. If developers or data scientists aren’t vigilant about the potential for bias, it can creep into the models they build.
An example of AI bias is racial discrimination in facial recognition software. Studies have shown these systems are significantly less accurate for women and people of colour compared to white men.
According to a study published by MIT Media Lab, error rates in determining the gender of light-skinned men were at 0.8 percent. However, for darker-skinned women, the error rates exceeded 20 percent in several cases. This is because these systems were predominantly trained on datasets that lacked sufficient diversity, leading to lower accuracy for non-white faces.
This bias in facial recognition technology has raised concerns about its use in policing and surveillance, where misidentification has severe consequences, as shown by a recent case in the UK.
To know more about this incident, read the full article.
Causes of AI bias
Historical inequality in data: Many datasets reflect past inequalities. For example, if a hiring dataset includes biased practices, the AI trained on it may perpetuate the same biases.
Skewed data representation: If certain groups are under or over represented in training data, the AI will learn to make predictions based on these imbalances. For instance, an AI model trained predominantly on images of light-skinned individuals may struggle to accurately recognise dark-skinned individuals as seen in Rober Williams' case.
Flawed data collection processes: The way data is collected can introduce bias. If data sources are incomplete, outdated, or collected with certain assumptions, the AI model may inherit these flaws. Imagine a council using AI to predict traffic patterns but collecting data only during weekdays. This dataset wouldn’t capture weekend traffic, leading the AI to make inaccurate predictions for those days. This happens not because of historical inequalities but because the data collection process missed important scenarios.
Unconscious bias of developers: Developers’ unconscious biases can influence how AI systems are designed, the data they prioritise, or the features they deem important. A lack of diversity among AI teams can also exacerbate this issue.
How to mitigate AI bias
Mitigating AI bias is critical not only for ethical reasons but also for the effectiveness and fairness of AI systems. Here are some strategies to help reduce or eliminate AI bias:
1. Diversify data sources
One of the first steps to reducing AI bias is ensuring that the data used to train models is diverse and representative of all groups. This means collecting data from a variety of sources and ensuring that under-represented groups are adequately included. For example:
- If you're developing an AI system to aid medical diagnoses, ensure that the training dataset includes a diverse set of patient profiles, covering different ethnicities, genders, ages, and medical conditions.
- In facial recognition, make sure the training data includes people from diverse racial and ethnic backgrounds, as well as different lighting conditions and angles.
2. Conduct bias audits and testing
Before deploying AI models in real-world applications, it’s essential to rigorously test them for bias. This involves running bias audits, where the AI model is examined to check if it disproportionately impacts certain groups.
- Implement techniques like adversarial testing, where AI systems are intentionally challenged with extreme or unusual scenarios to identify any biases.
- For example, testing a recruitment AI by submitting applications that represent diverse demographic groups, unusual career paths, or non-standard formats can uncover whether the system unfairly favours some or discriminates against specific characteristics.
- By proactively identifying and addressing such issues, organisations can reduce the risk of biased outcomes before deployment.
3. Implement transparency and explainability
AI models, particularly complex, deep learning systems, are often seen as "black boxes" because it can be difficult to understand how they arrive at a decision. Explainability helps developers, users, and stakeholders understand how AI decisions are made. Imagine applying for a loan and being denied, but you’re given no clear reason why. Was it your credit score, income, or something else entirely?
Explainability in AI ensures that when a decision is made—whether approving loans, diagnosing a medical condition, or screening job applications—developers and users can understand how and why the AI arrived at its conclusion.
- Transparent AI means that if a system makes a biased decision, it should be possible to trace the origin of the bias and correct it.
- This also helps build trust with the public, as individuals can better understand the logic behind AI decisions and spot potential sources of bias.
4. Incorporate ethical guidelines and human oversight
AI systems should not operate in isolation. Human oversight is essential, particularly in high-stakes areas like criminal justice or healthcare. Incorporating ethical guidelines during the design, training, and deployment of AI models can also help mitigate biases.
- Having diverse teams of developers, data scientists, and domain experts involved in the AI development process can bring different perspectives and reduce the likelihood of unconscious bias.
- Ethical frameworks, such as Australia's AI ethics and principles, proposed by the Department of Industry, Science and Resources, help ensure that AI systems respect fundamental rights, fairness, and accountability.
5. Regularly update and retrain models
AI models should not be static; they need to evolve as new data is collected and societal norms change. Regularly updating and retraining models with fresh, unbiased data can help ensure that AI systems stay fair and relevant.
- Feedback loops should be established so that users and stakeholders can flag instances of AI bias or unfair outcomes, which can then be addressed in subsequent iterations.
In addition to fairness, businesses must also prioritise privacy and security in AI systems to protect sensitive data. For a deeper dive into the critical considerations of privacy and security in AI adoption, check out our AI for business: Navigating privacy and security blog.
Final thoughts
AI has the potential to transform our world for the better, but its biases pose a significant threat to ensuring a fair and equal community. The responsibility falls on developers, policymakers, and society as a whole to ensure that AI evolves in a way that upholds fundamental principles of justice and ethics. Only through continued vigilance, education, and innovation can we hope to mitigate AI bias and unlock the full potential of these technologies.